Much of my professional life is now spent proselytizing: using practical examples I highlight the virtues of Bayesian inference, and warn against the intellectual Dementors that beset those who base conclusions on a p-value (yes, even when p<.005). And I am not alone: many Bayesians have a religious zeal to spread the faith and guide lost souls to the light. Nevertheless, not all researchers are Bayesian (yet). Why is this? Here I discuss eight arguments of why people may prefer to stay in Azkaban instead of switching to Bayesianism.
Eight Arguments for Azkaban
Recently my colleagues and I contributed two open-access articles on Bayesian inference for psychology, published in a special issue of Psychonomic Bulletin & Review. In Part I we outlined theoretical reasons for becoming a Bayesian, and in Part II we discussed practical applications of Bayesian inference using our free software package JASP. Part I starts by listing eight arguments that explain the lingering popularity of the p-value-based methodology:
“From a psychological perspective, however, a number of arguments may help explain the continued popularity of p values over Bayesian methods.1 First, researchers practice and preach the methodology that they were once taught themselves; interrupting this self-perpetuating educational cycle requires that researchers invest serious effort to learn new methods. Second, by breaking away from the dominant group of p value practitioners, researchers choose to move away from the in-group and expose themselves to the associated risks of academic exclusion. Third, just like fish form schools to escape predation, researchers may believe that there is security in repeating procedures that are popular; ‘surely,’ they may feel, ‘if the procedure I use is standard in the field, then any detractors must be overstating their case’. Fourth, many psychologists are primarily interested in addressing substantive research questions, not in the finer details of statistical methodology; such methodological disinterest feeds the desire for simple procedures that work well enough to convince the reviewers. In this sense the current p value fixation is similar to a statistical ritual (i.e., the ‘null ritual’, Gigerenzer, 2004). Fifth, the p value framework, when misinterpreted, offers a simple solution to deal with the uncertainty inherent in noisy data: when p < .05, reject H0 and accept H1; when p > .10, retain H0. When misapplied in this way, p values appear to make it easy for researcher to draw strong conclusions even when the empirical results are noisy and uninformative. Sixth, researchers may feel that by using non-standard methods (i.e., anything other than the p value) they reduce their chances of getting their work published or having it understood by their colleagues. Seventh, researchers interested in methodology have often internalized their statistical education to such an extent that they have difficulty accepting that the method they have used all their life may have serious limitations; when new information conflicts with old habits, the resulting cognitive dissonance can be reduced by discounting or ignoring the new information. Finally, it is possible that researchers may agree with the p value critiques, yet are unable to adopt alternative (Bayesian) inferential procedures. The reason for this inability is straightforward: virtually all statistical software packages produce p values easily, whereas Bayesian methods cannot count on the same level of support. Many of these arguments hold for statistical innovations in general, not just for p value NHST (Sharpe, 2013).
In general, then, powerful psychological and societal forces are at play, making it nigh impossible to challenge the dominant methodology. (Wagenmakers et al., 2018a, p. 36)
Lindley’s Perspective
We are hardly the first to speculate on the reasons why some researchers remain reluctant to move away from the p-value Azkaban. In a comment to Efron’s famous paper “Why isn’t everyone a Bayesian?”, Bayesian high-priest Dennis Lindley had made a suggestion similar to ours:
“The answer is simply that statisticians do not know what the Bayesian paradigm says. Why should they? There are very few universities in the world with statistics departments that provide a good course in the subject. Only exceptional graduate students leave the field of their advisor and read for themselves. A secondary reason is that the subject is quite hard for someone who has been trained in the sampling-theory approach to understand. (…) The subject is difficult. Some argue that this is a reason for not using it. But it is always harder to adhere to a strict moral code than to indulge in loose living. (…) Every statistician would be a Bayesian if he took the trouble to read the literature thoroughly and was honest enough to admit that he might have been wrong.” (Lindley, 1986, pp.6-7)
Cicero on the Attraction of Intellectual Azkabans
Two millennia ago, Cicero already noted that people, once they have been infected with a particular way of thinking, find it very hard to change gears and adopt a novel perspective:
“For all other people in the first place are held in close bondage placed upon them before they were able to judge what doctrine was the best, and secondly they form judgements about matters as to which they know nothing at the most incompetent period of life, either under the guidance of some friend or under the influence of a single harangue [i.e., diatribe – EJ] from the first lecturer that they attended, and cling as to a rock to whatever theory they are carried to by stress of weather. For as to their assertion that the teacher whom they judge to have been a wise man commands their absolute trust, I would agree to this if to make that judgement could actually have lain within the power of unlearned novices (for to decide who is a wise man seems to be a task that specially requires a wise man to undertake it); but granting that it lay within their power, it was possible for them after hearing all the facts and ascertaining the views of all the other schools as well, whereas they gave their verdict after a single hearing of the case, and enrolled themselves under the authority of a single master. But somehow or other most men prefer to go wrong, and to defend tooth and nail the system for which they have come to feel an affection, rather than to lay aside obstinacy and seek for the doctrine that is most consistent. [italics mine]” (Cicero, 45BC, Academica, II, 8-9; English translation by H. Rackham, 1933)
The journey ahead is long, but I have not given up hope and strongly believe that it will lead, inevitably and inexorably, to an escape out of the intellectual Azkaban of p-values.
Like this post?
Subscribe to the JASP newsletter to receive regular updates about JASP including the latest Bayesian Spectacles blog posts! You can unsubscribe at any time.
Footnotes
1 These arguments are speculative to the degree that they are based entirely on our personal experience and common-sense; in other words, our arguments have not been subjected to rigorous empirical tests.”
References
Cicero, M. T. (45 BC). Academica. English translation by H. Rackham, 1933.
Efron, B. (1986). Why isn’t everyone a Bayesian? The American Statistician, 40, 1-5.
Gigerenzer, G. (2004). Mindless statistics. The Journal of Socio-Economics, 33, 587-606.
Lindley, D. V. (1986). Comment on “Why Isn’t Everyone a Bayesian?” by Bradley Efron. The American Statistician, 40, 6-7.
Sharpe, D. (2013).Why the resistance to statistical innovations? Bridging the communication gap. Psychological Methods, 18, 572–582.
Wagenmakers, E.-J., Marsman, M., Jamil, T., Ly, A., Verhagen, A. J., Love, J., Selker, R., Gronau, Q. F., Smira, M., Epskamp, S., Matzke, D., Rouder, J. N., & Morey, R. D. (2018a). Bayesian inference for psychology. Part I: Theoretical advantages and practical ramifications. Psychonomic Bulletin & Review, 25, 35-57. Open Access.
Wagenmakers, E.-J., Love, J., Marsman, M., Jamil, T., Ly, A., Verhagen, A. J., Selker, R., Gronau, Q. F., Dropmann, D., Boutin, B., Meerhoff, F., Knight, P., Raj, A., van Kesteren, E.-J., van Doorn, J., Smira, M., Epskamp, S., Etz, A., Matzke, D., de Jong, T., van den Bergh, D., Sarafoglou, A., Steingroever, H., Derks, K., Rouder, J. N., Morey, R. D. (2018b). Bayesian inference for psychology. Part II: Example applications with JASP. Psychonomic Bulletin & Review, 25, 58-76. Open Access.
About The Author
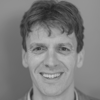