Redefine Statistical Significance Part XI: Dr. Crane Forcefully Presents…a Red Herring?
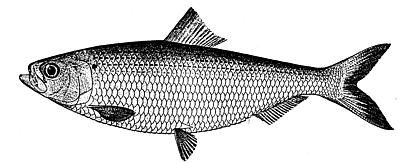
The paper “Redefine Statistical Significance” continues to make people uncomfortable. This, of course, was exactly the goal: to have researchers realize that a p-just-below-.05 outcome is evidentially weak. This insight can be painful, as many may prefer the statistical blue pill (‘believe whatever you want to believe’) over the statistical red pill (‘stay in Wonderland and see how deep the…
read more